Can We Democratize AI?
Can We Democratize AI?
Artificial intelligence has often been adopted in ways that reinforce exploitation and domination. But that doesn’t mean we should greet all new AI tools with refusal.
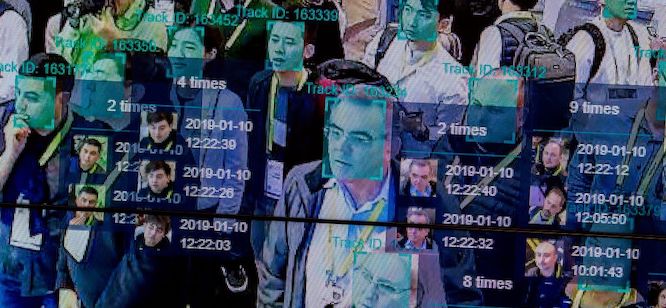
Atlas of AI
by Kate Crawford
Yale University Press, 2021, 336 pp.
At my suburban English secondary school in the early 1990s, sixteen-year-olds took a test to determine their optimal careers. After my exam, I was marched off to a two-week internship at an actuary’s office. The predictor foretold that I, too, would be a predictor, shuffling mortality data to compute debit and credit columns for an interminable future.
Even when I was a teenager, the idea of using historical data to make predictions was old hat. The Equitable Life Assurance Society, founded in 1762, underwrote risk by mining its own historical records. Starting in the 1830s, British firms used historical records to calculate fire insurance premiums. In 1903, New York Life adopted a nationwide insurance rating system using demographic and health data. By the 1950s, the Nielsen Company was collecting and exploiting television viewing data from hundreds of homes to predict future hits.
What now is known as “artificial intelligence” involves a similar process of informed guesswork, albeit with exponentially more data and harder math. AI-based prediction is now associated with the emergence of internet search, social-media platforms, the “gig” economy, and high-frequency trading. Prediction in this guise looms increasingly large in the economy. A UN document from 2019, for example, estimates that 71,966 Google searches are made globally every second.
Predictive AI also has an increasing number of policing, security, and military applications. As municipalities compete to become the leading “smart” city, and as welfare services are turned over to automated systems that facilitate their dissolution, the domain of state-driven machine prediction grows ever larger. On a grand scale, geostrategic rivalry with China drives increasing state subsidies for firms to craft new predictive tools and quantum computing to win a perceived race for AI dominance.
These changes are possible partly because the sheer volume of data available to crunch has dramatically increased since the 1970s, thanks to the diminishing marginal cost of processing and storing it. From the 1980s through the 2010s, breakthroughs in the design of the computational tools—most importantly, a process called backpropagation—opened the floodgates to a new breed of AI instruments. “Random forests,” “deep neural networks,” and “reinforcement learners” emerged to exploit the exabytes churned out by financial markets, social media, and internet use.
AI instruments are portrayed by boosters and critics alike as a tectonic technological breakthrough with major social, economic, and cultural implications. Some have argued that this amounts to a “second machine age,” not a mere variation on a theme familiar since the dawn of industrial capitalism. If this were true, it would follow that technology, not political economy, should be the central object of critical inquiry.
Kate Crawford starts with technology in Atlas of AI, but does not lose sight of its political economy. A leading figure in studies of new technologies, Crawford has cataloged AI’s misdeeds as co-founder of the AI Now Institute. In 2018, she created a startling and sobering digital map of the material resources, labor, data, and intellectual property used to create an Amazon Echo, which was later acquired by the Museum of Modern Art. In Atlas of AI, Crawford again raises economic-justice, privacy, and environmental concerns with AI practices. In her view, AI represents something new and malign because it has a singular, unambiguous, and fixed moral vector: It “is invariably designed to amplify and reproduce the forms of power it has been deployed to optimize.” Whatever social and economic arrangement is in place, she suggests, AI only makes it worse.
Atlas of AI builds on many predecessors. In an influential 2019 book The Age of Surveillance Capitalism, Shoshana Zuboff argued that the “surveillance economies” of Google and Facebook extract deeply personal and intimate data and use it to shape future behavior. Safiya Umoja Noble, Caroline Criado Perez, and Ruha Benjamin have all offered critiques of how AI tools amplify gender and racial bias. Antitrust scholars, led by Federal Trade Commission chair Lina Khan, have made the case that AI tools allow firms like Google and Amazon to suppress competition or sustain higher prices unlawfully.
Crawford braids together old and new critiques of AI into a larger story about technology as an instrument for sustaining hierarchy and exploitation. Innovations, in this account, should be analyzed through the power relations in which they are embedded. Echoing her past interdisciplinary work, Crawford blends history, political science, environmental science, and art (each chapter is prefaced by an elegant black-and-white image that encapsulates its themes). The result is a synoptic, poetic, and potent condemnation of AI in which Crawford responds directly and forcefully to the sunny writings emerging from the tech industry and its enthusiasts.
On the environmental front, where her narrative opens and ends, Crawford challenges the idea that Silicon Valley is a green economic sector without a heavy carbon footprint. Computers, she observes, require lithium and other rare resources. Deposits of lithium, such as one in Salar de Uyuni, in southwest Bolivia, have kindled sharp political tensions. The extraction of rare-earth minerals in the Democratic Republic of Congo fuels violent, destabilizing conflict. In both contexts, labor practices akin to slavery persist. At a commercial scale, Crawford notes, AI tools demand vast amounts of water to cool data centers. As machine-learning techniques have become more powerful, the amount of electricity needed to power them has risen exponentially.
Crawford also usefully details how AI facilitates labor exploitation. Echoing a theme familiar from books such as Martin Ford’s breathless Rise of the Robots, she contends that “corporations are heavily investing in automated systems in the attempt to extract ever-larger volumes of labor from fewer workers.” AI is thus built on the backs of Amazon’s factory workers, Mechanical Turkers, and content moderators who do piecemeal labor under grueling conditions with little compensation. Crawford is incensed by a particular corporate ideology advanced by AI boosters (she calls it a “hoax”), in which technology transcends concerns about the conditions of human labor.
And then there is the raw data that AI tools use to generate predictions. Facial-recognition systems—so controversial that cities such as San Francisco have banned them—are built on images from mugshot databases and government-issued IDs. These images are used, Crawford argues, by governments and firms without “input or consent” from the original subjects; these people are, as result “dehumanized” in some way. Again, she underscores the way AI boosters elide the origins of machine learning. Their talk of data as a “natural resource” hides more than it reveals.
If the problems with AI were only related to commercial exploitation, the remedy would be straightforward (if difficult to achieve): regulate or ban harmful practices. The problem, as Crawford recognizes, is that states also see advantages in using predictive technologies for domination and control. Edward Snowden’s disclosures about the NSA showed how metadata from telephone calls and emails could be mined to penetrate Americans’ privacy. More recently, the Department of Homeland Security partnered with Peter Thiel’s Palantir to build profiles of undocumented immigrants, including children, as tools for deportation. In China, development of a “social credit” system ranking citizens based on financial, political, and personal behavior points to new possibilities for state control of ordinary life.
For readers unfamiliar with the critical literature on AI, Crawford’s book provides a powerful, elegantly written synopsis. Her criticisms bite hard against the self-serving discourse of Silicon Valley. Yet I wonder whether her unqualified insistence that AI serves “systems that further inequality and violence” obscures at the same time as it illuminates. If data-based prediction, as I learned as a teenager, has been around a long time, how and when did it become such an irremediable problem?
More than a decade ago, the historian David Edgerton’s The Shock of the Old repudiated the notion that the future would be dematerialized, weightless, and electronic. Edgerton insisted on the endurance of old tools—diesel-powered ships carrying large metal containers, for example—as central components of neoliberal economic growth. It is a mistake, he suggested, to view our present deployments of technology as a function of innovation alone, free from the influence of inherited technological forms and social habits.
Crawford underscores pressing contemporary concerns about resource extraction, labor exploitation, and state violence. But has AI made these problems worse—or are current crises, as Edgerton’s analysis hints, just the enduring shock waves created by old technologies and practices? It’s not at all clear. Crawford, for instance, justly criticizes the energy consumption of new data centers, but she gives no accounting of the preceding history of data harvesting and storage unconnected to AI. As a result, it is unclear whether novel forms of AI have changed global rates of energy consumption and, if so, by what extent. Nor is it clear whether commercial AI is more or less amenable to reform than its predecessors. One of the leading scholarly articles on AI’s carbon footprint proposes a number of potential reforms, including the possibility of switching to already-available tools that are more energy efficient. And recent empirical work, published after Atlas of AI, shows that the energy consumption of major network providers such as Telefonica and Cogent decreased in absolute terms between 2016 and 2020, even as data demands sharply rose.
Similarly, Crawford’s analysis of the labor market for AI-related piecework does not engage with the question of whether new technologies change workers’ reservation wage—the lowest pay rate at which a person will take a particular kind of job—and hence their proclivity to take degrading and harmful work. It isn’t clear whether AI firms are lowering what is already a very lean reservation wage or merely using labor that would otherwise be exploited in a similar way. Crawford underscores the “exhausting” nature of AI-related labor—the fourteen-hour shifts that leave workers “totally numb.” But long, boring shifts have characterized capitalist production since the eighteenth century; we cannot know whether AI is making workers worse off simply by flagging the persistence of these conditions.
In Crawford’s narrative, AI is fated to recapitulate the worst excesses of capitalism while escaping even the most strenuous efforts at democratic regulation. Her critique of Silicon Valley determinism ends up resembling its photographic negative. Crawford here devotes few words to the most crucial question: can we democratize AI? Instead, she calls for a “renewed politics of refusal” by “national and international movements that refuse technology-first approaches and focus on addressing underlying inequities and injustices.”
It’s hard to disagree with the idea of fighting “underlying inequities and injustice.” But it’s not clear what her slogan means for the future use of AI. Consider the use of AI in breast-cancer detection. AI diagnostic tools have been available at least since 2006; the best presently achieve more than 75 percent accuracy. No difference has been observed in accuracy rates between races. In contrast, the (non-AI) pulse oximeter that found sudden fame as a COVID-19 diagnostic tool does yield sharp racial disparities. AI diagnostics for cancer certainly have costs, even assuming adequate sensitivity and specificity: privacy and trust may be lost, and physicians may de-skill. Returning to non-AI tools, though, will not necessarily eliminate “inequities and injustice.”
But do these problems warrant a “politics of refusal”? It would, of course, be a problem if accurate diagnostic AI were available only for wealthy (or white) patients; but is it a safe assumption that every new technology will reproduce extant distributions of wealth or social status? AI tools are often adopted under conditions that reinforce old forms of exploitation and domination or generate new ones. This is true of many technologies, however, from the cotton gin to the Haber-Bosch process. But cheaper cancer detection is just one of many possible examples of AI technologies that could expand the availability of services previously restricted to elites. An understanding of new AI technologies’ social potential demands not just Edgerton’s skepticism about novelty, that is, but an openness to ambiguity and contradiction in how tools can or should be used—a politics of progressive repossession, and not just refusal.
Crawford’s critiques of the damaging uses and effects of AI raise important questions about technological change under contemporary social conditions. Yet, by sidelining issues of historical continuity and the potential beneficial uses of new tools, she leaves us with an incomplete picture of AI, and no clear path forward. Atlas of AI begins a conversation then—but leaves plenty more to be said.
Aziz Z. Huq teaches at the University of Chicago. His book The Collapse of Constitutional Remedies was published in December 2021.